近日,華中農(nóng)業(yè)大學(xué)信息學(xué)院生物統(tǒng)計(jì)團(tuán)隊(duì)胡學(xué)海教授課題組研發(fā)出一款針對(duì)植物轉(zhuǎn)錄因子結(jié)合位點(diǎn)預(yù)測(cè)的工具及其docker鏡像,相關(guān)研究成果發(fā)表在國(guó)際生物信息學(xué)領(lǐng)域?qū)W術(shù)期刊Bioinformatics上。
轉(zhuǎn)錄因子結(jié)合位點(diǎn)(TFBS)是順式調(diào)控元件的基本組成部分,在基因表達(dá)的精確調(diào)控中起重要作用。TFBS核心基序內(nèi)的非編碼變異可能會(huì)顯著改變其結(jié)合親和力,這可能是解釋遺傳變異如何影響復(fù)雜性狀的生物學(xué)機(jī)制。植物中轉(zhuǎn)錄因子結(jié)合位點(diǎn)實(shí)驗(yàn)數(shù)據(jù)的缺乏,以及植物TFs的獨(dú)立進(jìn)化特性都使得鑒定植物TFBS的計(jì)算方法落后于相關(guān)的人類(lèi)研究。本研究首先使用深度卷積神經(jīng)網(wǎng)絡(luò)(DeepCNN)在基于可用的擬南芥Dap-seq數(shù)據(jù)集建立了265個(gè)擬南芥TFBS的預(yù)測(cè)模型,并且將其遷移用于預(yù)測(cè)其他植物的同源TF中。

建模結(jié)果表明,DeepCNN在265個(gè)擬南芥數(shù)據(jù)集上都獲得了很高的預(yù)測(cè)精確度(平均AUC達(dá)0.96),闡明了其在植物TFBS預(yù)測(cè)方面的可行性。通過(guò)進(jìn)一步深入分析DeepCNN中卷積核的性質(zhì),作者提供了模型的生物學(xué)可解釋性:DeepCNN不僅能學(xué)習(xí)到當(dāng)前轉(zhuǎn)錄因子在序列當(dāng)中的關(guān)鍵結(jié)合motif,而且能夠?qū)W習(xí)到與該轉(zhuǎn)錄因子共同協(xié)作的轉(zhuǎn)錄因子的結(jié)合motif。
最后當(dāng)使用遷移學(xué)習(xí)技術(shù)嘗試從計(jì)算的途徑解決目前植物TFBS研究問(wèn)題的困難時(shí),作者發(fā)現(xiàn)在不同的植物種類(lèi)中,遷移學(xué)習(xí)的表現(xiàn)具有很大的不同。在水稻的十個(gè)TF中的三個(gè)都取得了比較好的預(yù)測(cè)效果,BZIP23 、ERF48和MADS29的 PPV(Positive predictive value)分別為0.752、0.951和0.816。而當(dāng)遷移到玉米和大豆中時(shí),預(yù)測(cè)效果均不甚理想。這表明遷移學(xué)習(xí)在植物的跨物種轉(zhuǎn)錄因子結(jié)合位點(diǎn)預(yù)測(cè)問(wèn)題上具有一定的可行性,但是未來(lái)我們?nèi)孕柙O(shè)計(jì)更加有效的遷移學(xué)習(xí)策略。
為了提供更方便、更優(yōu)質(zhì)的生物信息學(xué)服務(wù),課題組為此具有高精確率辨別轉(zhuǎn)錄因子結(jié)合位點(diǎn)的深度卷積神經(jīng)網(wǎng)絡(luò)模型搭建了docker鏡像,通過(guò)下載該鏡像并在本地配置可以實(shí)現(xiàn)離線預(yù)測(cè)植物轉(zhuǎn)錄因子結(jié)合位點(diǎn)的預(yù)測(cè)功能(https://github.com/liulifenyf/TSPTFBS)。
【英文摘要】
Motivation: Both the lack or limitation of experimental data of transcription factor binding sites (TFBS) in plants and the independent evolutions of plant TFs make computational approaches for identifying plant TFBSs lagging behind the relevant human researches. Observing that TFs are highly conserved among plant species, here we first employ the deep convolutional neural network (DeepCNN) to build 265 Arabidopsis TFBS prediction models based on available DAP-seq (DNA affinity purification sequencing) datasets, and then transfer them into homologous TFs in other plants.
Results: DeepCNN not only achieves greater successes on Arabidopsis TFBS predictions when compared with gkm-SVM and MEME, but also has learned its known motif for most Arabidopsis TFs as well as cooperative TF motifs with PPI (protein-protein-interaction) evidences as its biological interpretability. Under the idea of transfer learning, trans-species prediction performances on ten TFs of other three plants of Oryza sativa, Zea mays and Glycine max demonstrate the feasibility of current strategy.
Availability and implementation: The trained 265 Arabidopsis TFBS prediction models were packaged in a Docker image named TSPTFBS, which is freely available on DockerHub at https://hub.docker.com/r/vanadiummm/tsptfbs. Source code and documentation are available on GitHub at: https://github.com/liulifenyf/TSPTFBS.
Contact: huxuehai@mail.hzau.edu.cn
原文鏈接:https://academic.oup.com/bioinformatics/article/37/2/260/6069568
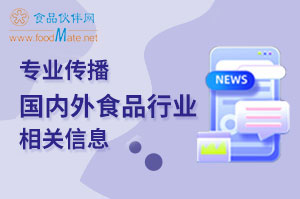